Case Study: Tree cavity habitats - Trametes
Year
2022 - ongoing
Location
7 sites in France
Deployment Size
100 devices
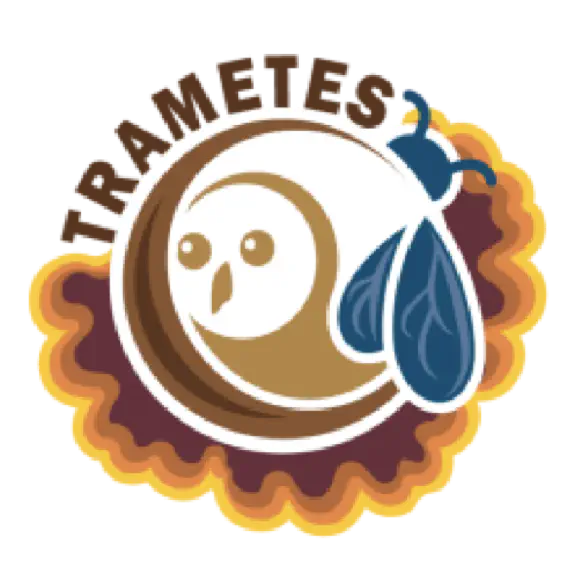
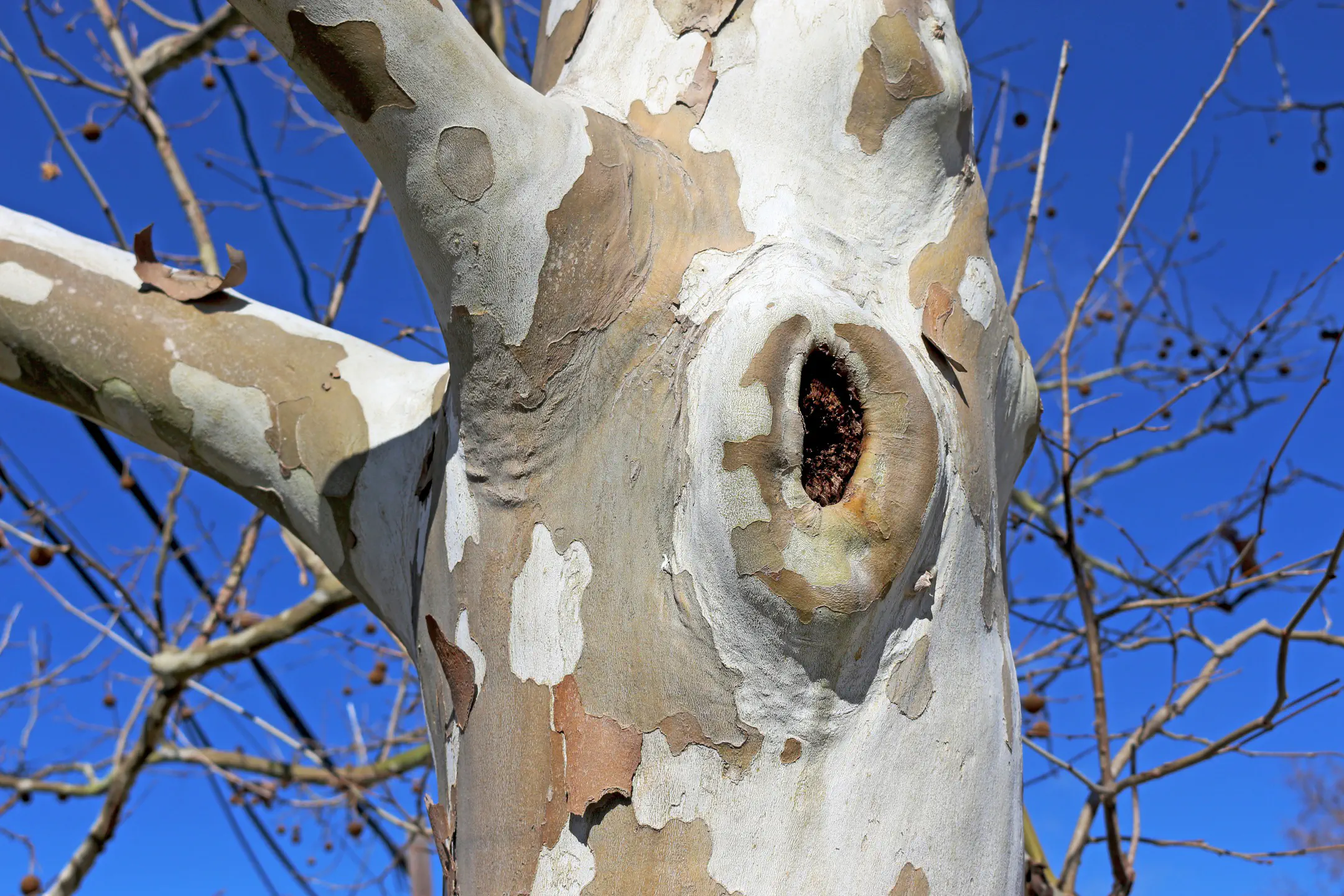
Habitat complexity is a crucial factor in promoting biodiversity, creating niches that enable specialized species to thrive (see Torres et al. 2020 ). In this project, we collaborated with a consortium led by scientist Yoan Paillet (INRAE, Grenoble) to study microdendrohabitats (tree cavities) and their role in supporting wild bees, bats, and birds. Our analysis involved quantifying and qualifying species populations, as well as examining habitat characteristics.
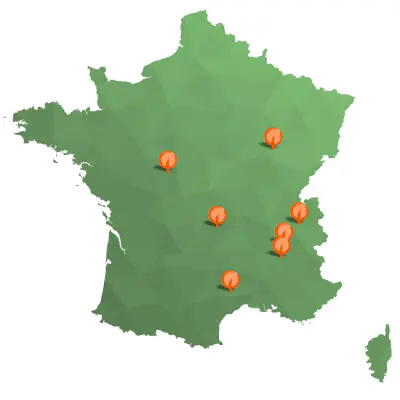
To accomplish this, we installed approximately 100 sensors across seven locations in France, comparing sites with known tree cavities against control sites. We employed distinct protocols for each species group, and the sensors are currently collecting data. While we conducted preliminary analyses on the already available data, more in-depth studies will follow in the coming years as a greater amount of data becomes available.
Problem statement
Tracking changes in bird, bee and bat populations over several months via monitoring devices, requires choosing effective measures that can capture these differences, while being on a constrained power budget. For this reason, the data collection protocol, including both sensing dimensions and scheduling, requires careful consideration to ensure that the project’s goals are met.
Approach and solution
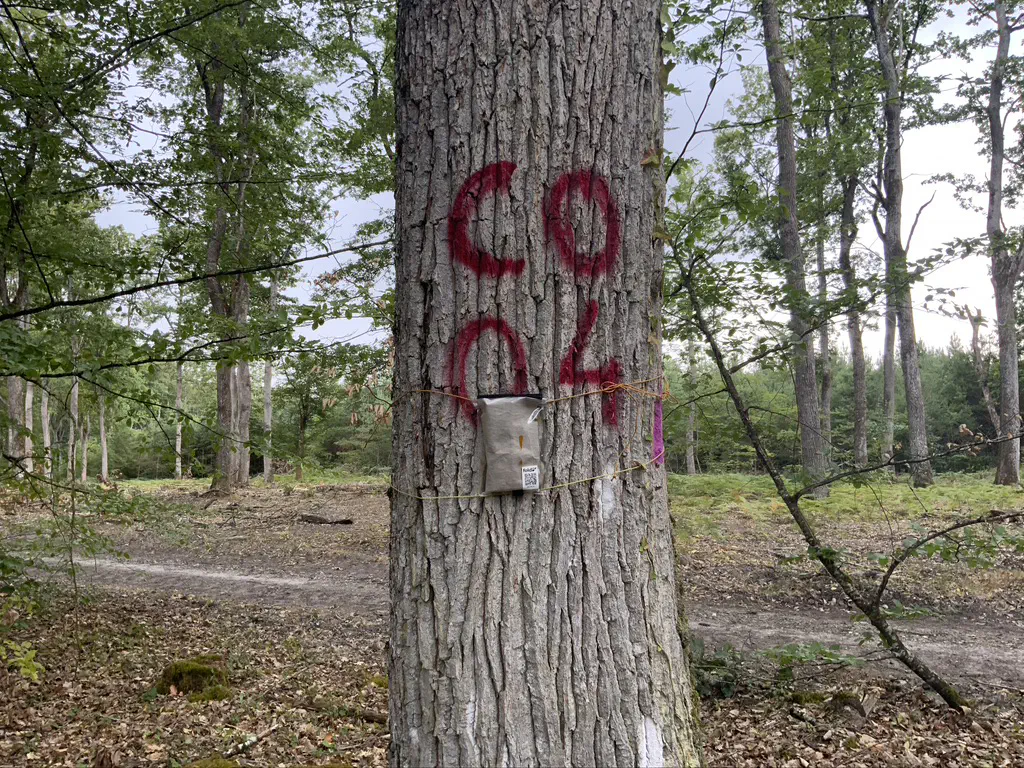
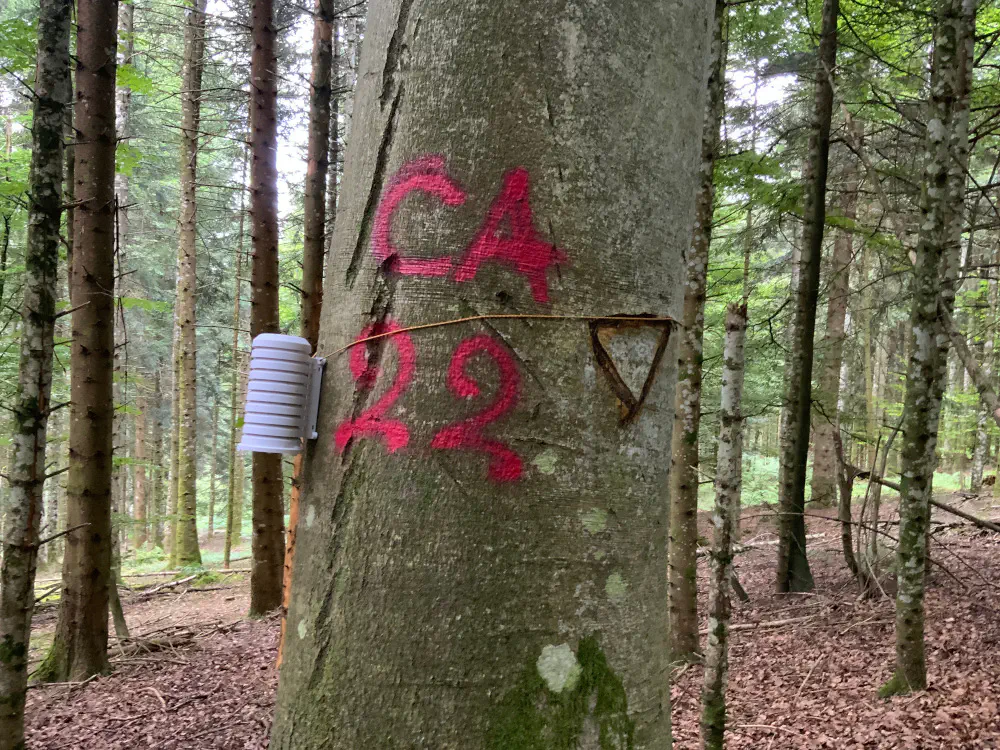
Our approach to addressing the issue of monitoring bees, birds, and bats involved designing custom data recording protocols for our Aja devices. These protocols involved the capture of raw audio data for all three species, including ultra-sonic frequencies for bats. Additionally, we recorded tree trunk vibrational patterns for bee activity, to gather further insight into their behavior. To ensure reliable data collection, we installed and regularly maintained the sensors at the test sites.
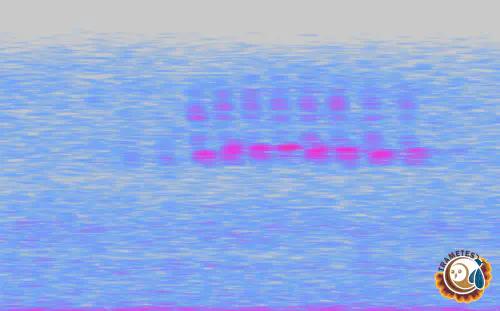
For the preliminary data analysis, we used a combination of automatic and manual techniques to extract valuable insights. For example, we utilized tools like BirdNet to automatically classify bird species based on their recorded vocalizations, and other signal processing & Machine Learning techniques to detect bat activity. The project also saw our data scientists digging through the data to gain a deeper understanding of the behavior and patterns of the monitored species.
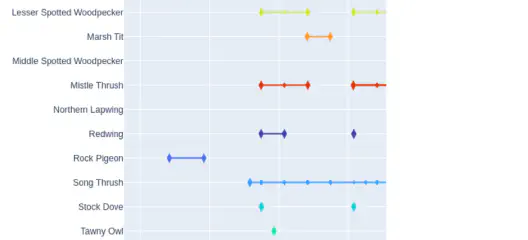
To be able to tackle this project’s challenged, our team brought forwards several innovations in our devices’ and data infrastructure, including
- high-resolution raw data acquisition,
- novel ultra-sound recording capabilities for the detection of bats, and tree trunk vibrational analysis for the monitoring of bee activity
- NB-IoT connectivity for device telemetry data,
- extending our data pipeline, to store and analyze the multiple terabytes of audio data produced by the devices.